Inappropriateness in visual media is deeply woven into the human condition – machines need to accommodate for different cultural, legal and social frameworks in order to become better tools for content moderation and safety
OULU, FINLAND – Publishers, broadcasters, and online content providers in particular, are struggling to keep up with the sheer amount of content and ways to manage it. This problem manifests strongly in the fast-paced social media platforms, and is causing a new headache for companies that manage high volumes of content, as highlighted by the Wall Street Journal . The surge of generated visual media, video and images, has created a new business need that is being met by innovative companies utilizing AI to profoundly understand what’s inside the content – and help identifying the unwanted parts.
Simple AI-based video and image moderation tools have been around for some time now and they have turned into good companions in spotting well-defined concepts like nudity, weapons and swastikas. However, our engagement with businesses working with visual media has taught us that there are a lot of parameters that go into defining what is appropriate from the cultural, legal and social perspectives. The context created with visual compositions in media has a huge impact on what is considered inappropriate from the perspective of brands and publishers. This is why early recognition solutions have often been considered insufficient in providing satisfactory solution for automated moderation and more sophisticated solutions are very much in demand. A revealing outfit can signify different levels of appropriateness across cultures and social groups.
The challenge of building an AI that recognizes content appropriateness is vastly more complex problem than e.g. building a simple classifier to categorize cat vs dog pictures. Why? There are at least three forces in play. Firstly, the contextual differences in visual pattern representations are very large within the population of inappropriate content, and the level of variety in training examples needs to be large even for singular concepts, like recognizing the differences between occluded nudity and full nudity.
Second, the challenge of predicting if any piece of visual content stems from the inappropriate content domain is a challenge of recognizing data concepts in the wild setting, where managing what an inappropriate concept is, is equally important to managing what the concept is not as there is no way of controlling the extent and variety of the input data. A good case study for building cognitive application in the wild setting can be read from Tim Anglade’s blog post on building a recognition app for hot dogs as depicted in HBO’s Silicon Valley series.
Third, the legal, regional and cultural aspects in defining what is appropriate makes it very difficult to rely on training singular concept models, like classifying adult vs non-adult content for different media market regions. Being adult-oriented is a moving target and needs to be adaptable to different social situations. Moreover, in programmatic advertising brands want to have a safe online inventory to protect their brands. A scalable solution for different needs is to plan a broad visual moderation taxonomy carefully with grounds in objective rather than subjective representations.
Valossa AI updated for better visual moderation and compliance
For the past months, we have been developing a large update to our inappropriate video and image recognition models, and the latest update in February reflects the months of work we have poured into development of proper compliance and moderation tool. Our goals were to build a cognitive service that (a) recognizes nuanced variations between the extreme and milder versions of phenomena, and (b) increases in the wild reliability and concept coverage for variety of pattern representations through carefully moderated taxonomy and extensive AI training for any media content.
By significant effort, we trained Valossa AI to cope with the variety of real, context-dependent situations in images and videos:
- Separating between knife in a cooking context vs a person threatening with a knife;
- Separating bomb explosions vs other natural disasters;
- Separating male vs female sensual content, frontal nudity vs nudity from behind, and more.
The key approach that we used was detailing a taxonomy that covers a variety of elements that constitute an inappropriate content entity. With our technology global media companies have more automated control over variety of content types and styles – and can adapt to different local criteria for age restrictions, broadcast times and viewer discretion.
Moreover, we wanted to discover to what extent other cognitive service providers can cope with more nuanced content. For that, we devised a benchmark with inappropriate media content and ran through several different cognitive moderation vendors, focusing here on the services from Amazon, Google, Microsoft and IBM, the Big Four cloud service vendors.
The expected function of visual content moderation service is not only to predict inappropriate or not, but to explain the inappropriateness for more nuanced validation
We benchmarked how major vendors perform in more fine-grained adult or inappropriate categories.
To test the services against the premise described above, we put together a benchmark image test consisting of images from 16 different content moderation and compliance categories in typical media (e.g. movies and series) and ran them through different vendors.
To succeed, a cognitive service should be able to score detections in all categories: like winning a decatohectothlon (yes, it really is a word meaning sports contest with 16 events). Poor performance in individual categories would result in a lower overall ranking.
The categories were: lifeless body, blood/gore/open wound, aiming with a gun, hand-to-hand combat, melee weapons and combat, smoking, drug use, alcohol, explicit nudity, sexual activity, sensual content, guns and weapons, destruction, explosions, flood, and traffic accident.
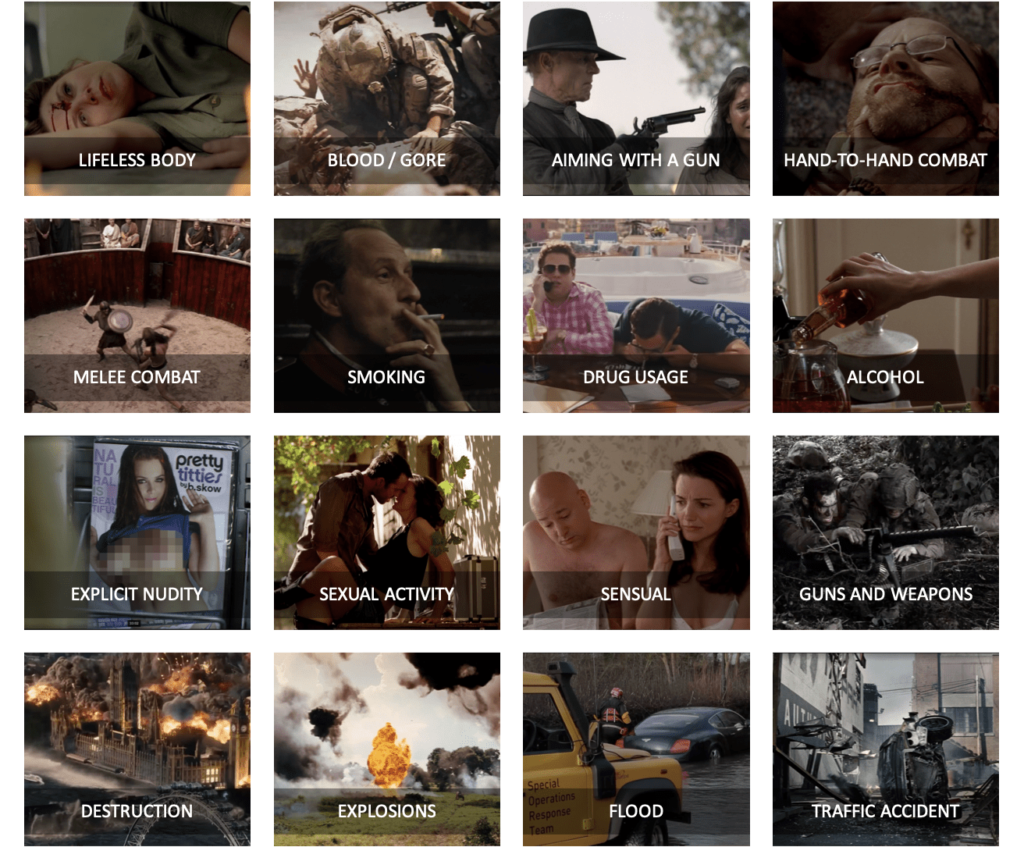
The dataset of 162 images containing inappropriate visuals was processed through each cognitive service, and recall performance was measured, i.e. how many true positive predictions were made and how many were left undetected. Recall is the more important performance metric for moderation, since low sensitivity leads to false negatives, and that can turn out expensive for business. Vendors were scored based on their recall performance using ordinal ranks 1-8 in each category and the accumulated rank score average was calculated to measure overall performance in the peer group of services.
According to the test, Valossa AI reached the best overall recall rank score against the measured cognitive cloud services. We particularly highlight Amazon Rekognition, Google Cloud Vision, IBM Watson and Microsoft Azure Computer Vision as the reference performance we managed to beat with our careful approach on training visual moderation AI. You might be thinking that you should take these results with a grain of salt, and we are happy to answer any questions you have about the experiments. We chose to use 0.5 as the level of confidence across all providers, but we acknowledge this is an approximation and comprehensive precision-recall curves would be the more robust approach to find out about different sensitivity-specificity ranges between all systems.
Across all providers, most difficult categories to recognize were hand-to-hand combat, substance use, and destruction. Easiest categories were about sex, nudity and guns. As can be imagined, context plays more important role in the depiction of substance use and fist fights rather than full frontal nudity, therefore being more complex to train for the AI. Valossa AI is continuously being updated with these challenging categories and improvements are rolled out every month to our public service.
Finally, in addition to visual moderation in videos, there’s another modality that is important to consider in content safety use cases: speech. Valossa AI video moderation contains also speech transcription and monitoring of bad language words for multiple languages.
All things considered, we believe our design approach around comprehensiveness and nuanced taxonomy coverage has taken the industry an important step forward towards semi and fully automated video moderation systems. We encourage companies working on image and video moderation tasks to start testing our latest models.
If you are intrigued in trying out Valossa AI for content moderation, you can either
- Start immediately by playing around with our image recognition demo including moderation tags
- Sign-up at Valossa Portal to start experimenting with our video recognition platform including interactive audiovisual compliance reporting tool, and Video recognition API access
- Contact our sales (sales@valossa.com) if you want to start developing your applications using our image recognition APIs
Contact
Lauri Penttilä
Marketing & Communications Manager
lauri.penttila@valossa.com